The Healthcare CMO's Roadmap to AI-Driven Patient Journey Segmentation
Healthcare marketing has undergone a fundamental transformation. Gone are the days of broad demographic targeting and one-size-fits-all campaigns. Today's healthcare consumers expect personalized experiences that anticipate their needs at every stage of their health journey. For enterprise healthcare CMOs, AI-powered segmentation represents not just an evolution in marketing technology, but a strategic imperative.
In an industry where the stakes include patient outcomes and care continuity, segmentation isn't merely about driving conversions—it's about delivering the right information to the right patient at the right moment in their healthcare journey. This level of precision requires moving beyond traditional models toward AI-driven approaches that recognize complex behavioral patterns and predict future needs.
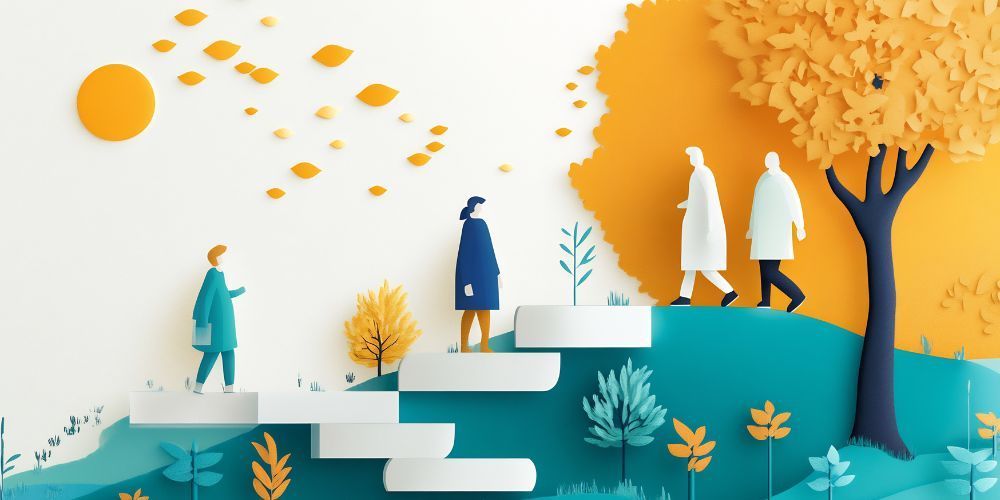
Why Traditional Segmentation Falls Short in Healthcare
Traditional segmentation in healthcare typically follows predictable patterns:
- Demographic grouping (age, gender, location)
- Basic medical history categorization
- Insurance coverage classification
- General service line interests
While these approaches have served as foundational elements, they fail to capture the nuanced reality of modern patient journeys. A 58-year-old male in Boston with similar demographic and medical profiles to his peers may have entirely different:
- Digital channel preferences
- Information-seeking behaviors
- Decision-making timelines
- Trust factors with providers
- Care access challenges
AI-powered segmentation addresses these limitations by identifying patterns in vast datasets that would be impossible for human analysts to detect. This enables healthcare organizations to move from segmenting based on static attributes to dynamic, behavior-based modeling that evolves with the patient.
The Strategic Value Proposition of AI Segmentation
For healthcare CMOs, AI segmentation delivers tangible strategic value:
- Improved Marketing ROI: Cleveland Clinic implemented AI-driven segmentation for their cardiovascular service line and saw a 34% increase in qualified leads with a 22% reduction in cost per acquisition.
- Enhanced Patient Acquisition: Northwell Health's AI segmentation initiative identified previously overlooked behavioral indicators that predicted interest in telehealth services, resulting in 28% higher conversion rates for their virtual care offerings.
- Strengthened Patient Retention: Kaiser Permanente's AI-powered retention program identified at-risk patients based on subtle engagement patterns, allowing for timely interventions that improved retention rates by 17%.
- Better Resource Allocation: Mayo Clinic's precision segmentation initiative helped redistribute marketing spend from overserved segments to high-potential growth areas, improving overall marketing efficiency by 26%.
When properly implemented, AI segmentation becomes more than a marketing tactic—it transforms into a strategic asset that drives enterprise value and improves patient outcomes simultaneously.
The Four Stages of AI Segmentation Maturity in Healthcare
Healthcare organizations typically progress through four stages of AI segmentation sophistication:
Stage 1: Foundational
Characteristics:
- Enriched basic demographics with behavioral data
- Rules-based segmentation with limited automation
- Single-channel focus (typically email)
Example application: Automatically categorizing patients into broad groups like "preventive care seekers" or "chronic condition managers" based on appointment history and portal activity.
Typical tools:
- Salesforce Health Cloud with basic Einstein features
- Microsoft Dynamics 365 Healthcare Accelerator
- Basic implementations of Marketo or Eloqua
Stage 2: Advanced
Characteristics:
- Multichannel behavioral tracking
- Predictive modeling for next best actions
- Integration of clinical and marketing data (within compliance boundaries)
Example application: Predicting which communication channels and content types will most effectively engage different patient segments based on their past interactions and preferences.
Typical tools:
- Salesforce Health Cloud with advanced Einstein AI
- Persado for AI-generated content variations
- Adobe Experience Cloud with healthcare-specific models
- SAS Customer Intelligence
Stage 3: Transformational
Characteristics:
- Real-time segment adjustment based on behavior
- Propensity modeling for service line interests
- Journey-based segments rather than static groups
- Integration with operational systems for seamless experiences
Example application: Dynamic segmentation that adapts as patients move through different life stages or health conditions, automatically adjusting content and channel strategy in real-time.
Typical tools:
- Amperity Customer Data Platform with healthcare models
- Actium Health's CENTARI platform
- Innovaccer Healthcare Data Platform
- Epsilon PeopleCloud Health
Stage 4: Prescriptive
Characteristics:
- Hyper-personalized 1:1 experiences at scale
- Prescriptive recommendations for next-best-experience
- Autonomous optimization across all touchpoints
- Closed-loop analytics with clinical outcome correlation
Example application: Fully autonomous marketing systems that continuously optimize messaging, timing, channel, and content based on individual patient needs, preferences, and health conditions while maintaining strict HIPAA compliance.
Typical tools:
- PathFactory with healthcare content intelligence
- OneSpot personalization platform
- Darwin AI by Persado
- Hyros for healthcare
- Linus Health's digital platform
Most enterprise healthcare organizations currently operate between Stages 1 and 2, with leading innovators pushing into Stage 3. The progression through these stages isn't just technological—it requires corresponding advancements in strategy, organization, and governance.
Building Your AI Segmentation Roadmap
Step 1: Audit Current Segmentation Capabilities
Before investing in new technology, assess your current state:
- Inventory existing data sources and integration points
- Evaluate current segmentation models and their effectiveness
- Identify key performance gaps in patient engagement
- Assess your team's analytical capabilities and AI readiness
- Review compliance frameworks and data governance policies
Key question: Where in the four-stage maturity model does your organization currently operate?
Step 2: Define Clear Business Objectives
AI segmentation must solve specific business problems:
- Are you primarily focused on new patient acquisition?
- Do you need to improve retention for specific service lines?
- Is patient education and adherence a primary concern?
- Are you working to shift low-acuity care to digital channels?
- Do you need to better coordinate marketing with value-based care initiatives?
Key question: What measurable outcomes will define success for your AI segmentation initiative?
Step 3: Develop Your Data Strategy
Effective AI segmentation depends on data quality and integration:
- Determine what first-party data you currently collect and its quality
- Identify gaps in your patient journey data
- Develop a strategy for appropriate data enrichment within compliance boundaries
- Create a roadmap for breaking down data silos across departments
- Implement robust data governance with special attention to PHI considerations
Key question: What are your critical data gaps, and how will you address them while maintaining HIPAA compliance?
Step 4: Select the Right Technology Partners
The healthcare MarTech landscape is complex—your choices should align with your strategic objectives:
- For patient data management: Consider healthcare-specific CDPs like Innovaccer, Actium Health, or Welltok
- For marketing automation: Evaluate Salesforce Marketing Cloud, Adobe Experience Cloud, or Microsoft Dynamics 365 Marketing
- For predictive analytics: Look at healthcare-tailored solutions from SAS Healthcare, Evariant, or healthcare modules from Tableau/Power BI
- For personalization engines: Evaluate OneSpot, Evergage, or Dynamic Yield with healthcare configurations
Key question: Which vendors have demonstrated success with organizations at a similar stage in the maturity model?
Step 5: Build an Implementation Roadmap
Develop a phased approach to implementation:
- Pilot phase (3-6 months): Focus on a single service line with clear KPIs
- Expansion phase (6-12 months): Apply learnings to 2-3 additional service lines
- Integration phase (12-18 months): Connect AI segmentation with operational systems
- Transformation phase (18+ months): Scale across the enterprise and move toward prescriptive capabilities
Key question: What quick wins can demonstrate value while building toward the long-term vision?
Step 6: Address Organizational Readiness
Technology alone won't drive success. Prepare your organization:
- Develop AI literacy programs for marketing team members
- Create new roles or upskill existing talent for AI-driven marketing
- Establish cross-functional teams spanning marketing, IT, analytics, and clinical
- Implement governance frameworks that balance innovation with compliance
- Develop change management strategies to drive adoption
Key question: What skills, roles, and organizational structures need to evolve to support AI-driven segmentation?
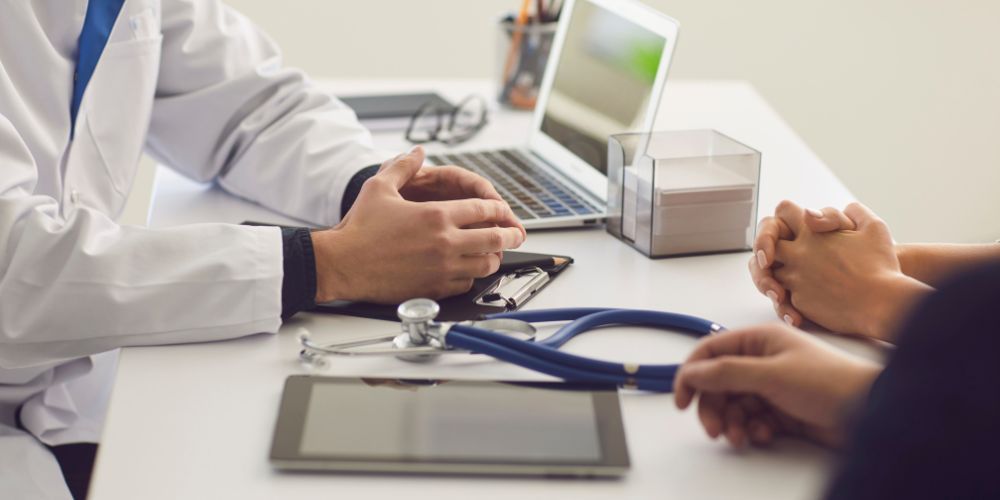
Implementation Case Study: Stanford Health Care
Stanford Health Care's journey provides a blueprint for enterprise healthcare organizations:
Challenge: Stanford needed to better engage patients across its complex network of specialty and primary care services while supporting its shift toward value-based care models.
Approach:
- Created a unified patient data foundation by integrating marketing data with approved clinical data
- Implemented Actium Health's CENTARI platform to identify behavioral patterns
- Developed AI models to predict which patients would benefit from preventive screenings
- Created dynamic content journeys based on predicted care needs
- Implemented closed-loop measurement connecting marketing activities to care outcomes
Results:
- 36% increase in preventive screening appointments
- 28% reduction in patient acquisition costs
- 42% improvement in campaign conversion rates
- Measurable contribution to value-based care metrics
Key insight: Stanford's success came not just from the technology, but from the close collaboration between marketing, clinical leaders, and analytics teams to develop meaningful segmentation models that aligned with both business and patient care objectives.
Measuring Success: KPIs for AI-Powered Segmentation
Traditional marketing metrics remain important, but AI segmentation enables more sophisticated measurement.
Tactical Metrics:
- Segment-specific engagement rates
- Channel preference accuracy
- Content affinity prediction accuracy
- Conversion rate by AI-defined segment
Strategic Metrics:
- Patient lifetime value by segment
- Cross-service line utilization
- Care plan adherence rates
- Preventive care adoption
- Contribution to value-based care metrics
Operational Metrics:
- Campaign deployment efficiency
- Time-to-insight for new segments
- Model accuracy and refinement rates
- Team productivity with AI assistance
Common Pitfalls and How to Avoid Them
As you implement AI-driven segmentation, be aware of these common challenges.
1. Neglecting Ethical Considerations
Pitfall: Implementing AI without addressing bias, transparency, and privacy concerns.
Solution: Establish an AI ethics framework specifically for healthcare marketing that addresses bias testing, explainability requirements, and extra safeguards for vulnerable populations.
2. Technology-First Thinking
Pitfall: Investing in sophisticated AI tools without clear use cases or organizational readiness.
Solution: Start with business problems, not technology solutions. Define clear objectives and use cases before selecting technology partners.
3. Insufficient Cross-Functional Collaboration
Pitfall: Marketing operating in isolation from clinical, IT, and compliance teams.
Solution: Create formal structures for collaboration between marketing, IT, analytics, clinical leadership, and compliance from the earliest planning stages.
4. Data Quality and Integration Challenges
Pitfall: Building models on incomplete, inaccurate, or siloed data.
Solution: Invest in data quality and integration before scaling AI segmentation. Start with a focused data set you can trust rather than trying to include everything.
5. Expecting Immediate Results
Pitfall: Abandoning initiatives when they don't show immediate ROI.
Solution: Set realistic timelines with early milestone metrics. AI segmentation typically shows incremental improvements over 6-12 months before delivering transformational results.
The Future of AI Segmentation in Healthcare
Looking ahead, several trends will shape the evolution of AI segmentation.
1. Longitudinal Health Journey Mapping
Future AI models will move beyond episodic care segmentation to understand lifetime health journeys, enabling healthcare marketers to develop decades-long relationship strategies with patients.
2. Integrated Physical-Digital Experience Orchestration
AI segmentation will bridge physical and digital touchpoints, creating seamless experiences whether a patient is on a website, mobile app, in a waiting room, or during a telehealth visit.
3. Value-Based Care Alignment
Segmentation models will increasingly connect marketing activities directly to value-based care priorities, demonstrating marketing's contribution to clinical and financial outcomes.
4. Cross-Organizational Data Collaboration
Healthcare systems, payers, and wellness providers will create collaborative data environments that enable more holistic views of the patient journey while maintaining privacy compliance.
5. Zero-Party Data Emphasis
As privacy regulations tighten, AI models will place greater emphasis on explicitly provided patient preferences and intentions rather than inferred behaviors.
From Marketing Tactic to Strategic Asset
For enterprise healthcare CMOs, AI-powered segmentation represents more than a marketing innovation—it's a strategic asset that bridges marketing and clinical objectives. Organizations that successfully implement these capabilities gain a significant competitive advantage: the ability to deliver relevant, timely, and meaningful experiences throughout the patient journey.
The most successful healthcare marketing leaders approach AI segmentation not as a technology implementation but as a strategic transformation that touches every aspect of patient engagement. By following the roadmap outlined in this guide, you can move beyond basic demographics toward a future where every patient interaction is informed by intelligent insights, aligned with care needs, and designed to build lasting relationships.
How does AI segmentation differ from traditional RFM (Recency, Frequency, Monetary) models in healthcare marketing?
Traditional RFM models in healthcare classify patients based on their past behavior—when they last engaged with your health system, how often they seek care, and the financial value of services they've utilized. While useful for basic segmentation, these models have significant limitations in healthcare.
AI segmentation fundamentally differs by incorporating predictive elements rather than just historical behavior. Instead of simply categorizing patients based on past actions, AI models identify complex patterns that predict future health needs, channel preferences, and response likelihood. For example, an AI model might recognize that patients who engage with certain educational content and exhibit specific portal behavior patterns are likely to need specialty care referrals in the next 3-6 months.
The key difference is that AI segmentation operates dynamically, continuously recategorizing patients based on their evolving health journey rather than placing them in static buckets. This enables healthcare marketers to move from reactive to proactive engagement strategies that anticipate patient needs before they're explicitly expressed.
What HIPAA considerations should healthcare CMOs prioritize when implementing AI segmentation?
HIPAA compliance must be foundational to any AI segmentation strategy. Start by establishing clear boundaries between PHI (Protected Health Information) and marketing data. Work with compliance officers to define explicit rules for what patient data can be used for marketing purposes and under what circumstances.
1. Prioritize these key considerations:
Consent management: Implement granular consent processes that clearly communicate how patient data will be used and allow patients to control their preferences.
2. De-identification protocols: Develop robust processes for properly de-identifying clinical data before it enters marketing systems, ensuring compliance with the Safe Harbor or Expert Determination methods outlined in HIPAA.
3. Purpose limitations: Establish clear boundaries for how AI-derived insights can be used, documenting specific marketing use cases approved by your compliance team.
4. Vendor assessment: Thoroughly evaluate the HIPAA compliance of all MarTech vendors, with particular attention to how AI models are trained and where data is stored.
5. Access controls: Implement role-based access controls that limit which team members can view different types of segmentation data.
6. Documentation: Maintain detailed records of your data flows, segmentation logic, and compliance reviews to demonstrate due diligence.
Many leading healthcare organizations establish a dedicated governance committee with representatives from marketing, IT, legal, and clinical leadership to review and approve AI segmentation use cases before implementation.
How should we organize our marketing team to maximize the value of AI-powered segmentation?
Successfully implementing AI segmentation requires rethinking traditional healthcare marketing team structures. Rather than organizing primarily by channel (email team, social team, etc.) or service line, consider a hybrid model that balances specialized AI expertise with business context.
Most effective healthcare organizations adopt one of these approaches:
1. Center of Excellence Model: Create a dedicated AI marketing team that serves as internal consultants to traditional marketing functions. This team typically includes data scientists, ML engineers, and marketing technologists who develop and maintain segmentation models while training the broader organization on their application.
2. Embedded Expertise Model: Distribute AI specialists across existing marketing teams, embedding data scientists within service line marketing groups. This approach accelerates adoption but requires strong central governance to maintain consistent standards.
3. Hybrid Partnership Model: Maintain a core AI team for infrastructure and model development while training "AI translators" within each marketing function who can bridge technical capabilities with business needs.
Regardless of the model, successful teams typically include these emerging roles:
- Marketing Data Scientists who specialize in healthcare behavioral analysis
- AI Ethics Officers who ensure segmentation models avoid bias and comply with regulations
- Patient Journey Architects who design personalized content journeys based on AI insights
- Segmentation Strategists who translate AI insights into actionable marketing approaches
The most successful transformations also involve significant investment in upskilling existing team members, with particular focus on analytical thinking, data interpretation, and basic model understanding rather than just technical skills.