A Strategic Guide to AI-Powered Audience Segmentation
Beyond Basic Demographics: How B2B CMOs Can Deploy AI for Hyper-Targeted Segmentation
In the complex B2B landscape, understanding your audience has never been more critical—or more challenging. Traditional segmentation based on firmographics like company size, industry, and location still has its place, but today's most successful B2B organizations are leveraging artificial intelligence to develop deeper, more nuanced audience insights that drive meaningful engagement and accelerate revenue growth.
As B2B buying committees expand (now averaging 6-10 decision-makers across multiple departments) and buying journeys become increasingly non-linear, marketing leaders need more sophisticated approaches to audience segmentation. This is where AI is transforming what's possible.
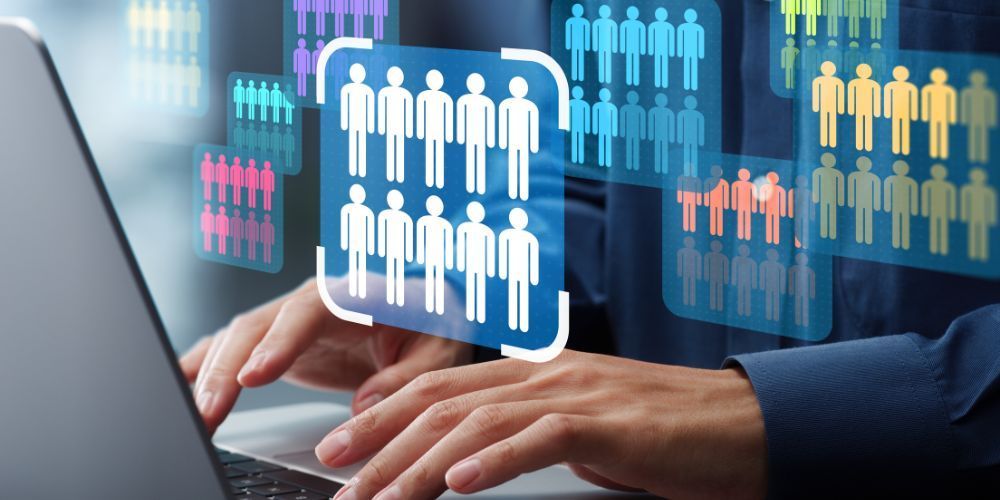
The Evolution of B2B Audience Segmentation
From Firmographics to Behavioral Intelligence
Traditional B2B segmentation has followed a predictable path:
- Firmographic Segmentation: Company size, industry, location, revenue
- Demographic Segmentation: Job titles, departments, seniority
- Technographic Segmentation: Technology stacks, software adoption
- Intent-Based Segmentation: Content consumption, search behavior
Each approach delivered incremental improvements, but all were constrained by the same fundamental limitation: they relied on historical data patterns that humans could identify and interpret. AI changes this paradigm entirely by uncovering hidden patterns and correlations that human analysts might never discover.
How AI Transforms B2B Audience Segmentation
Moving Beyond Human-Defined Categories
AI-powered segmentation fundamentally differs from traditional approaches in three ways:
- Dynamic vs. Static: Traditional segments remain relatively stable; AI-driven segments evolve continuously based on new behavioral data.
- Multi-dimensional vs. Linear: Where traditional segmentation might consider 3-5 variables, AI can analyze hundreds of data points simultaneously to create complex, multi-dimensional segments.
- Predictive vs. Descriptive: Traditional segmentation describes who customers are; AI-powered segmentation predicts what they'll do next.
The result? B2B marketers can move from "educated guesses" about audience needs to data-driven certainty, dramatically improving campaign performance and resource allocation.
Building Your AI Segmentation Foundation
Step 1: Audit Your Data Infrastructure
Before implementing AI for audience segmentation, you need to evaluate your data foundation. Consider:
- Data Completeness: Do you have sufficient first-party data across the customer journey? Are there significant gaps in your understanding of customer behavior?
- Data Integration: Can you connect behavioral data across marketing platforms, sales interactions, and customer support touchpoints?
- Data Quality: How clean and consistent is your data? Are there duplicate records or inconsistent formatting that would undermine analysis?
For most B2B organizations, enhancing data quality and integration will be necessary prerequisites for effective AI implementation. This often requires:
- Implementing consistent data collection standards across platforms
- Establishing unified customer identifiers across touchpoints
- Creating processes for regular data cleansing and validation
Step 2: Define Business Objectives
AI-powered segmentation is not an end in itself but a means to achieving specific business outcomes. Before diving into implementation, clearly define what you aim to accomplish, such as:
- Increasing conversion rates among high-value prospects
- Reducing sales cycle length for specific customer types
- Improving customer retention and expansion in target accounts
- Optimizing resource allocation across marketing channels
This clarity will guide your choice of AI approaches and success metrics.
Step 3: Select Your AI Approach
Different business objectives may require different AI techniques. Common approaches include:
- Clustering Algorithms: Identify natural groupings in your customer base without predefined categories
- Predictive Modeling: Forecast which accounts are most likely to convert, expand, or churn
- Natural Language Processing: Analyze unstructured data from sales calls, support tickets, and social media
- Deep Learning: Identify complex patterns across massive datasets that simpler algorithms might miss
Most B2B organizations benefit from starting with clustering algorithms and basic predictive models before advancing to more sophisticated approaches.
Four Strategic Applications of AI-Powered Segmentation in B2B
1. Account Prioritization Through Propensity Modeling
In B2B marketing, all potential customers are not created equal. AI can help identify which accounts have the highest probability of converting by analyzing:
- Historical conversion patterns across similar accounts
- Current engagement signals across marketing and sales touchpoints
- External factors like funding announcements, leadership changes, and growth indicators
This enables marketing and sales teams to focus resources on accounts with the highest revenue potential rather than spreading efforts evenly across all prospects.
Implementation Strategy
- Begin with a supervised learning model trained on historical conversion data
- Incorporate CRM data, marketing automation engagement metrics, and third-party intent signals
- Create a scoring system that sales and marketing teams can easily interpret and act upon
- Continuously refine the model based on actual conversion outcomes
2. Content Journey Optimization
B2B buying journeys typically involve extensive research and evaluation. AI can help identify which content assets are most effective at moving specific audience segments through their decision process by:
- Mapping content consumption patterns to pipeline progression
- Identifying content gaps that slow down the buying process
- Recommending personalized content journeys based on role, industry, and past engagement
Implementation Strategy
- Implement comprehensive content tagging and tracking
- Analyze content consumption patterns across different customer segments
- Use machine learning to identify successful content sequences that lead to conversion
- Develop automated content recommendation engines for your website, email, and sales enablement platforms
3. Buying Committee Mapping
B2B purchases involve multiple stakeholders with different priorities, information needs, and objections. AI can help identify and map these buying committees by:
- Analyzing engagement patterns to identify multiple stakeholders within the same account
- Inferring roles based on content consumption and interaction behavior
- Tracking relationship dynamics among committee members
This intelligence enables more strategic account-based marketing that addresses the needs of each stakeholder rather than treating accounts as monolithic entities.
Implementation Strategy
- Develop machine learning models that identify engagement patterns indicating committee involvement
- Create visualization tools that help sales teams understand buying committee structures
- Design targeted messaging strategies for each committee member based on their inferred priorities
4. Behavior-Based Customer Expansion Targeting
For many B2B companies, the greatest growth opportunity lies in expanding relationships with existing customers. AI can identify expansion opportunities by:
- Detecting usage patterns that indicate readiness for additional products or services
- Identifying accounts with similar characteristics to those that have previously expanded
- Highlighting customer behavior that suggests potential churn risk, enabling proactive intervention
Implementation Strategy
- Train models on historical expansion data to identify leading indicators
- Implement real-time monitoring of customer usage metrics and engagement signals
- Develop automated alerting systems that notify account teams of expansion opportunities or churn risks
- Create personalized expansion journeys based on product usage and engagement
Implementation Challenges and Solutions
Challenge 1: Data Silos and Integration Issues
Most B2B organizations store customer data across multiple systems: CRM, marketing automation, customer success platforms, billing systems, and more. This fragmentation creates significant barriers to effective AI implementation.
Solution: Implement a customer data platform (CDP) or similar integration layer that creates unified customer profiles across systems. Prioritize API-driven architectures that enable real-time data synchronization.
Challenge 2: Algorithm Transparency and User Adoption
AI-driven segmentation can appear as a "black box" to marketing and sales teams, creating resistance to adoption when they don't understand how segments are determined.
Solution: Invest in explainable AI approaches that provide clear rationales for segmentation decisions. Create intuitive visualizations and interfaces that help business users understand and trust AI-driven insights.
Challenge 3: Balancing Personalization and Privacy
As segmentation becomes more granular, organizations must navigate growing privacy concerns and regulations, particularly when using third-party data.
Solution: Develop clear data governance frameworks that prioritize first-party data collection. Implement privacy-by-design principles in your segmentation strategy, including clear consent mechanisms and data minimization practices.
Challenge 4: Maintaining Human Oversight
AI excels at identifying patterns in historical data but may miss emerging trends or contextual factors that human marketers would recognize.
Solution: Implement hybrid approaches that combine AI-driven segmentation with human expertise. Establish regular review processes where marketing teams can evaluate and refine AI-generated segments based on their market knowledge.
Measuring Success: Beyond Traditional Metrics
The value of AI-powered segmentation should be measured not just by marketing metrics but by its impact on overall business outcomes. Key metrics to consider include:
- Pipeline Velocity: Average time from first engagement to closed deal
- Win Rate Improvements: Conversion rate changes by segment
- Customer Lifetime Value: Changes in expansion and retention by segment
- Resource Efficiency: Marketing and sales cost per acquisition
- Predictive Accuracy: How well the models predict actual customer behavior
Most importantly, establish clear baseline measurements before implementing AI segmentation so you can accurately assess impact.
Getting Started: A Practical Roadmap
For B2B CMOs looking to implement AI-powered segmentation, I recommend a phased approach:
Phase 1: Foundation Building (Months 1-3)
- Audit existing data quality and integration
- Establish clear business objectives and success metrics
- Implement necessary data collection and integration tools
- Begin building unified customer profiles
Phase 2: Pilot Implementation (Months 4-6)
- Select a high-impact use case for initial implementation
- Build and train your first AI models using historical data
- Implement a small-scale pilot with a subset of accounts
- Measure results and refine approach
Phase 3: Expansion and Optimization (Months 7-12)
- Scale successful approaches across additional segments
- Implement more sophisticated AI techniques
- Integrate AI insights with marketing execution platforms
- Establish ongoing optimization processes
Phase 4: Advanced Implementation (Year 2+)
- Implement real-time segmentation capabilities
- Develop fully automated personalization systems
- Explore predictive analytics for future market trends
- Integrate customer and market intelligence
The Future of AI-Powered Segmentation
As AI capabilities continue to evolve, B2B segmentation will become increasingly:
- Real-time: Segments will update instantly based on behavioral signals
- Predictive: Models will forecast future needs with greater accuracy
- Autonomous: AI will not just identify segments but automatically optimize engagement
- Conversational: Natural language interfaces will enable marketers to query and interact with segmentation insights
Organizations that build strong AI segmentation foundations today will be best positioned to leverage these emerging capabilities.
From Segmentation to True Understanding
The most significant promise of AI-powered segmentation isn't just more precise targeting—it's a fundamentally deeper understanding of customer needs and behaviors. By uncovering patterns and correlations that would remain hidden in traditional analysis, AI helps B2B organizations move from simply classifying customers to truly understanding them.
This deeper understanding enables not just more effective marketing but more customer-centric product development, more intuitive user experiences, and more valuable customer relationships. In an increasingly competitive B2B landscape, this level of customer understanding isn't just a marketing advantage—it's a business imperative.
The organizations that thrive in the coming decade won't be those with the most data or the most advanced algorithms, but those that most effectively translate AI-driven insights into meaningful customer experiences and business value.
Do we need to hire data scientists to implement AI-powered segmentation for our B2B marketing?
Not necessarily. While having data expertise is valuable, many modern marketing platforms now offer built-in AI capabilities that don't require specialized data science skills. Start by evaluating your current MarTech stack for AI functionalities you may already have access to. For more advanced applications, consider partnering with specialized agencies or consultants who can guide implementation without requiring full-time data scientists. The most important first step is organizing your data infrastructure properly—a task that often requires more business logic than advanced data science.
How can we measure the ROI of implementing AI-powered segmentation in our B2B marketing?
Measuring ROI from AI segmentation requires tracking both direct campaign improvements and broader business impacts. Start by establishing clear baselines for key metrics like conversion rates, sales cycle length, and customer acquisition costs before implementation. Then track improvements across multiple dimensions: marketing efficiency (reduced cost per qualified lead), sales effectiveness (higher win rates, faster sales cycles), and customer outcomes (increased retention, larger deal sizes). The most compelling ROI typically comes from comparing the performance of AI-identified high-potential segments against traditional targeting approaches. Document both quantitative improvements and qualitative benefits like sales team satisfaction with lead quality.
Our B2B data is limited or messy. Can we still benefit from AI-powered segmentation?
Yes, but with a staged approach. Start by focusing on data quality rather than quantity. Clean, consistent data across a few key dimensions will yield better results than vast amounts of unreliable information. Begin with your most complete data sets—typically CRM and marketing automation data—and implement AI segmentation in one specific area, such as identifying which existing customers have the highest expansion potential. As you demonstrate value and improve data collection practices, gradually expand your AI segmentation initiatives. Consider enriching your first-party data with third-party sources to fill critical gaps, but prioritize building better internal data collection processes for long-term success.